Data Engineering on Microsoft Azure
Course ID : DP-203T00-A
In this course, the student will learn about the data engineering as it pertains to working with batch and real-time analytical solutions using Azure data platform technologies. Students will begin by understanding the core compute and storage technologies that are used to build an analytical solution. The students will learn how to interactively explore data stored in files in a data lake. They will learn the various ingestion techniques that can be used to load data using the Apache Spark capability found in Azure Synapse Analytics or Azure Databricks, or how to ingest using Azure Data Factory or Azure Synapse pipelines. The students will also learn the various ways they can transform the data using the same technologies that is used to ingest data. They will understand the importance of implementing security to ensure that the data is protected at rest or in transit. The student will then show how to create a real-time analytical system to create real-time analytical solutions.
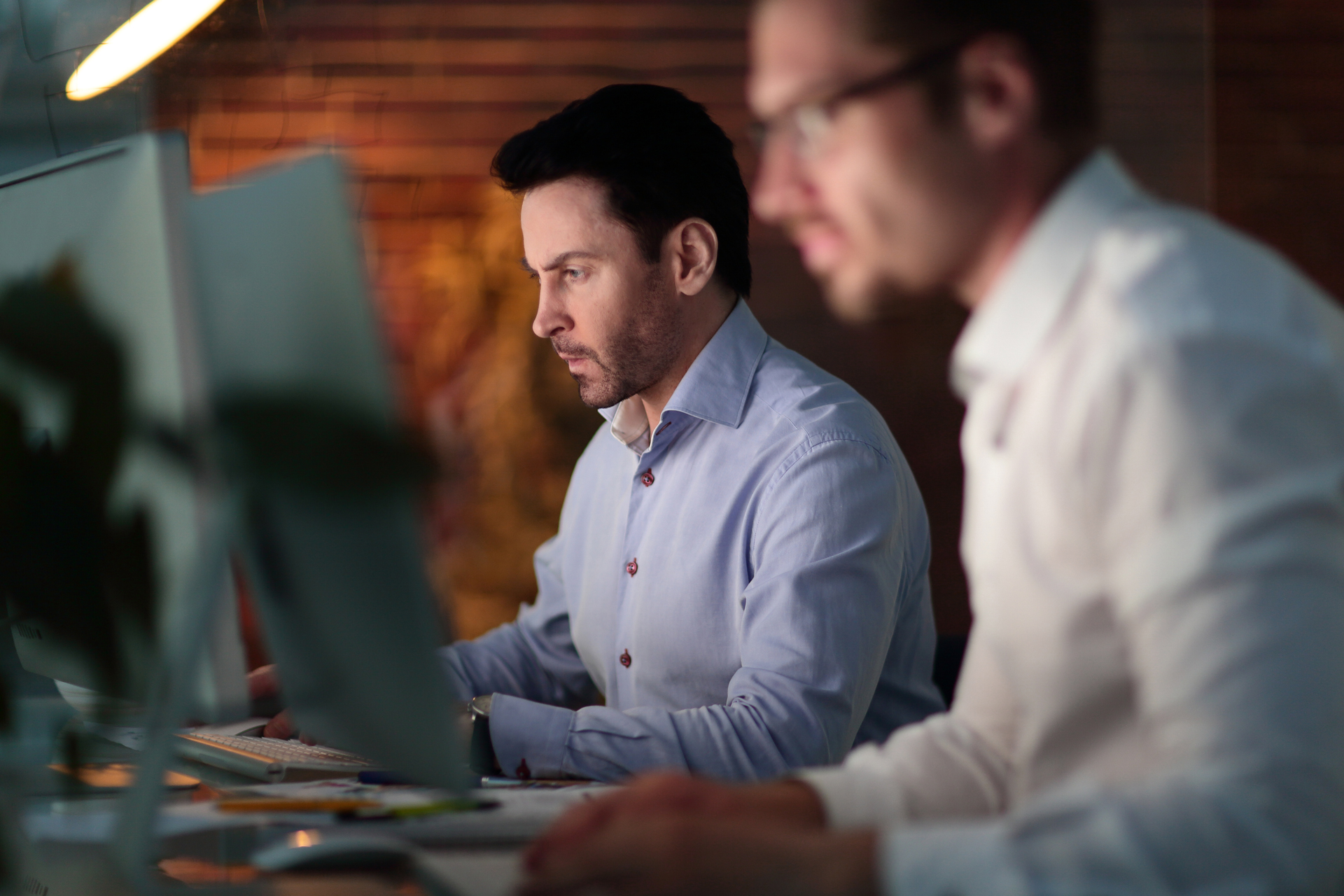
- 4 Days
- Intermediate
- Tunisia / Bahrain
- English / French
- Virtual & Classroom Training
- Certification: N/A
- 4 Days
- Intermediate
- Tunisia / Bahrain
- English / French
- Virtual & Classroom Training
- Certification: N/A
Module 1: Explore compute and storage options for data engineering workloads
- Introduction to Azure Synapse Analytics
- Describe Azure Databricks
- Introduction to Azure Data Lake storage
- Describe Delta Lake architecture
- Work with data streams by using Azure Stream Analytics
- Lab 1: Explore compute and storage options for data engineering workloads
- Combine streaming and batch processing with a single pipeline
- Organize the data lake into levels of file transformation
- Index data lake storage for query and workload acceleration
Module 2: Run interactive queries using Azure Synapse Analytics serverless SQL pools
- Explore Azure Synapse serverless SQL pools capabilities
- Query data in the lake using Azure Synapse serverless SQL pools
- Create metadata objects in Azure Synapse serverless SQL pools
- Secure data and manage users in Azure Synapse serverless SQL pools
- Lab 2: Run interactive queries using serverless SQL pools
- Query Parquet data with serverless SQL pools
- Create external tables for Parquet and CSV files
- Create views with serverless SQL pools
- Secure access to data in a data lake when using serverless SQL pools
- Configure data lake security using Role-Based Access Control (RBAC) and Access Control List
Module 3: Data exploration and transformation in Azure Databricks
- Describe Azure Databricks
- Read and write data in Azure Databricks
- Work with DataFrames in Azure Databricks
- Work with DataFrames advanced methods in Azure Databricks
- Lab 3: Data Exploration and Transformation in Azure Databricks
- Use DataFrames in Azure Databricks to explore and filter data
- Cache a DataFrame for faster subsequent queries
- Remove duplicate data
- Manipulate date/time values
- Remove and rename DataFrame columns
- Aggregate data stored in a DataFrame
Module 4: Explore, transform, and load data into the Data Warehouse using Apache Spark
- Understand big data engineering with Apache Spark in Azure Synapse Analytics
- Ingest data with Apache Spark notebooks in Azure Synapse Analytics
- Transform data with DataFrames in Apache Spark Pools in Azure Synapse Analytics
- Integrate SQL and Apache Spark pools in Azure Synapse Analytics
- Lab 4: Explore, transform, and load data into the Data Warehouse using Apache Spark
- Perform Data Exploration in Synapse Studio
- Ingest data with Spark notebooks in Azure Synapse Analytics
- Transform data with DataFrames in Spark pools in Azure Synapse Analytics
- Integrate SQL and Spark pools in Azure Synapse Analytics
Module 5: Ingest and load data into the data warehouse
- Use data loading best practices in Azure Synapse Analytics
- Petabyte-scale ingestion with Azure Data Factory
- Lab 5: Ingest and load Data into the Data Warehouse
- Perform petabyte-scale ingestion with Azure Synapse Pipelines
- Import data with PolyBase and COPY using T-SQL
- Use data loading best practices in Azure Synapse Analytics
Module 6: Transform data with Azure Data Factory or Azure Synapse Pipelines
- Data integration with Azure Data Factory or Azure Synapse Pipelines
- Code-free transformation at scale with Azure Data Factory or Azure Synapse Pipelines
- Lab 6: Transform Data with Azure Data Factory or Azure Synapse Pipelines
- Execute code-free transformations at scale with Azure Synapse Pipelines
- Create data pipeline to import poorly formatted CSV files
- Create Mapping Data Flows
Module 7: Orchestrate data movement and transformation in Azure Synapse Pipelines
- Orchestrate data movement and transformation in Azure Data Factory
- Lab 7: Orchestrate data movement and transformation in Azure Synapse Pipelines
- Integrate Data from Notebooks with Azure Data Factory or Azure Synapse Pipelines
Module 8: End-to-end security with Azure Synapse Analytics
- Secure a data warehouse in Azure Synapse Analytics
- Configure and manage secrets in Azure Key Vault
- Implement compliance controls for sensitive data
- Lab 8: End-to-end security with Azure Synapse Analytics
- Secure Azure Synapse Analytics supporting infrastructure
- Secure the Azure Synapse Analytics workspace and managed services
- Secure Azure Synapse Analytics workspace data
Module 9: Support Hybrid Transactional Analytical Processing (HTAP) with Azure Synapse Link
- Design hybrid transactional and analytical processing using Azure Synapse Analytics
- Configure Azure Synapse Link with Azure Cosmos DB
- Query Azure Cosmos DB with Apache Spark pools
- Query Azure Cosmos DB with serverless SQL pools
- Lab 9: Support Hybrid Transactional Analytical Processing (HTAP) with Azure Synapse Link
- Configure Azure Synapse Link with Azure Cosmos DB
- Query Azure Cosmos DB with Apache Spark for Synapse Analytics
- Query Azure Cosmos DB with serverless SQL pool for Azure Synapse Analytics
Module 10: Real-time Stream Processing with Stream Analytics
- Enable reliable messaging for Big Data applications using Azure Event Hubs
- Work with data streams by using Azure Stream Analytics
- Ingest data streams with Azure Stream Analytics
- Lab 10: Real-time Stream Processing with Stream Analytics
- Use Stream Analytics to process real-time data from Event Hubs
- Use Stream Analytics windowing functions to build aggregates and output to Synapse Analytics
- Scale the Azure Stream Analytics job to increase throughput through partitioning
- Repartition the stream input to optimize parallelization
Module 11: Create a Stream Processing Solution with Event Hubs and Azure Databricks
- Process streaming data with Azure Databricks structured streaming
- Lab 11: Create a Stream Processing Solution with Event Hubs and Azure Databricks
- Explore key features and uses of Structured Streaming
- Stream data from a file and write it out to a distributed file system
- Use sliding windows to aggregate over chunks of data rather than all data
- Apply watermarking to remove stale data
- Connect to Event Hubs read and write streams
Audience(s)
- Data professionals
- Data architects
- Business intelligence professionals
Objectives
- Design and implement data storage on Azure
- Design and develop data processing on Azure
- Design and implement data security on Azure
- Monitor and optimize data storage and data processing on Azure
Related Course
Associated Badge
Data Engineering on Microsoft Azure
Course ID : DP-900T00-A
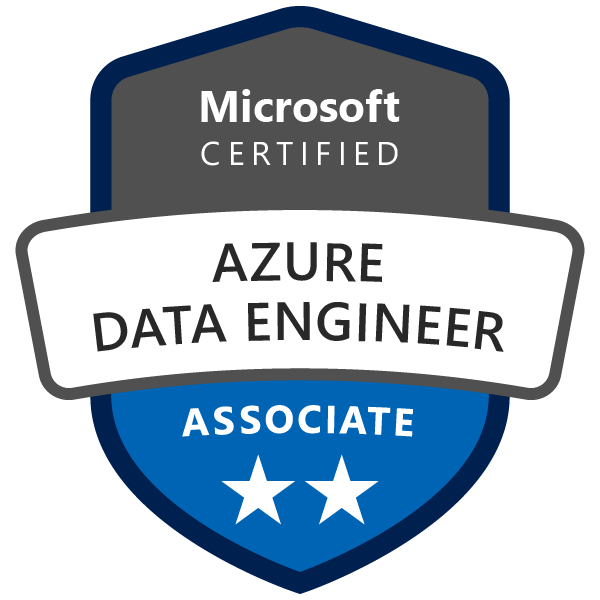
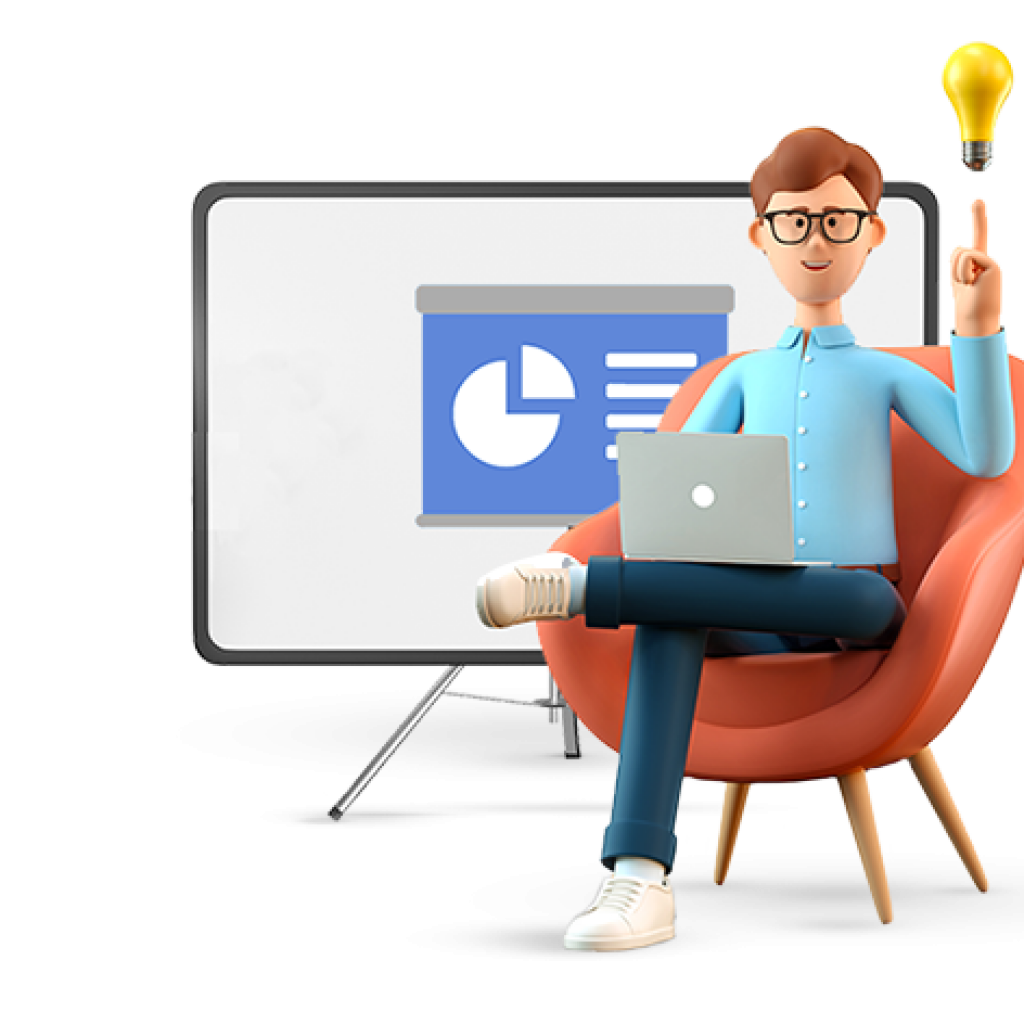
Ready for the training ? Fill the form
Make the most of your online learning experience
Our Online Learning Resource Center has tips, tricks and inspiring stories to help you learn while staying home.
THINK
Grow your expertise and advance your career